Introduction
“Big data” is already a frequently-heard buzzword, both in the business analytics arena, but also in the field of high-performance scientific computing. Basically, “big data” encompasses the collection, processing, indexing and utilization of large-scale datasets. Some concrete examples include temperature and sunlight data downloaded from satellites monitoring of the Earth’s environment, particle tracking data produced by the Large Hadron Collider in Europe, and anonymized smartphone position data made available, in some cases, by wireless operators and even certain smartphone applications.
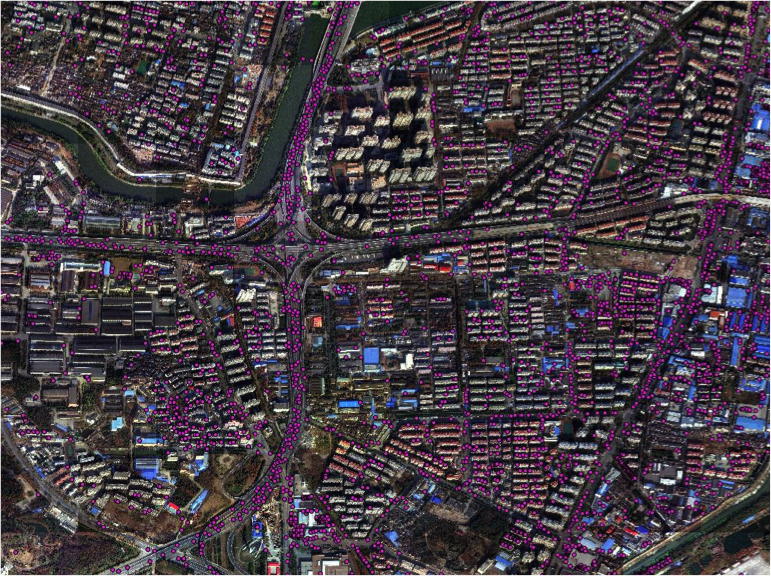
Courtesy Quandl, DigitalGlobe and Orbital Insight
Big data has enormous potential to revolutionize the world of finance, mainly because of its potential to enable human investors and their machine-learning computer counterparts to spot economic trends.
The key idea here is that with such data in hand, big-data-equipped investment operations, such as quantitative hedge funds, may be able to spot trends even before the industries directly involved are aware of them. Indeed, we may well be seeing the day when quarterly reports or even press reports are relegated to the realm of “old news,” with their content already known (and acted upon) hours, days or even weeks earlier by those equipped with big data tracking tools.
Big data in action
Big data is already being applied in the area of finance. For example, in 2015 certain hedge funds utilizing satellite data sources noted rising traffic in the parking lots of J.C. Penny stores, and were able to beat other investors to the punch. Indeed, JCP’s stock jumped more than 10% when public reports of JCP’s increased store traffic came to light in August.
As another example, in 2015 some investment firms were able to conclude that U.S. corn production was 2.8% smaller than prevailing government estimates, based on analysis of infrared satellite images taken of over one million corn fields.
Other types of big data include shopping mall traffic, coal shipments, oil storage tanks, industrial plant production, flood data, ship location data, mobile payments and even geo-tagged smartphone traffic.
Such feats do not come easy, even if one uses, say, public data from NASA’s Landsat satellite system. For example, to accurately predict crop yields, photos must be monitored for several years, keeping close tabs on many individual patches of lands. Then such data must be correlated with data on the type of crop (e.g., corn or soybeans), date of germination, and typical yield. Noting the difference in appearance between when a field has produced a high yield compared with a lower yield, machine-learning techniques must then be employed to more accurately predict current crop yields.
Principal players
There are numerous players in the field, including the following:
- Planet Labs has deployed nearly 100 “cube sats,” shoebox-sized (10cm x 10cm x 30cm) satellites that continuously scan the Earth and send data whenever one passes over a ground station. Planet provides their clients with 3-5 meter resolution, updated frequently.
- DigitalGlobe offers both satellite images and machine-learning software to enable customers to glean insights from its library.
- Planet IQ focuses on weather and climate modeling.
- Orbital Insight focuses on analytic software for satellite and other remote sensing data, even including synthetic aperture radar (SAR) data. They claimed in 2016 that since 2013, their US Retail Traffic Index predicted a beat or miss of Bloomberg consensus estimates 78% of the time.
- Descartes Labs, which was spun off from the Los Alamos National Laboratory, has noted successes in predicting changes in domestic corn production, based on changes in plant color over time. They have plans to extend their reach to drones and mobile phones.
- RS Metrics, which features data in retail traffic, real estate, metals production and others.
- Spire, which focuses on ships, planes and weather.
AI and Data Science Conference in New York
The Artificial Intelligence and Data Science: Capital Markets conference, organized by Newsweek, just concluded in New York City. It reviewed a number of these developments, and discussed some additional issues.
One concern was the matter of privacy. Jonathan Streeter, of Dechert LLP, who has focused on legal and regulatory aspects of big data for several years, warned that those who employ data, particularly in the finance world, need to be careful. Although, as one might expect, governmental regulation has lagged significantly behind these fast-breaking technological developments, nonetheless there is a prevailing notion that users of big data have an obligation to perform “due diligence,” double-checking that several levels of privacy protection have been satisfactorily dealt with: Did the purveyors of such data properly obtain permission from their sources for subsequent usage? If the data originated with consumers, did the form that they completed properly disclose such third-party usage? If anything, users of big data should bend over backwards to avoid privacy controversies that could derail much beneficial usage.
There is also an overriding concern in several quarters that increasing reliance on artificial intelligence and big data might render markets too brittle to handle the next major downturn. Robert Kaplan, the president of the Dallas Federal Reserve Bank, recently raised concern that the present-day regime of relatively low volatility is “extraordinarily unusual.” Thus AI-big data systems may actually exacerbate the next correction, as the era of low volatility and relatively cheap money comes to an end. Indeed, it is not clear how these systems will react during a shock event.
Conclusion
We pointed out in a previous Math Investor blog that pretty much all of the hedge funds that have consistently beaten the market averages in recent years are those that employ highly mathematical, data-intensive strategies. This was underscored in a new Bloomberg article, which reported additional closures among traditional hedge funds, yet quantitative funds such as Renaissance Technologies and Two Sigma are still attracting new funds and clients.
It may well be seen, in retrospect, that this movement to highly quantitative, data-intensive investing is merely the first step in the sort of high-powered machine-learning and big data analysis described above.
So, is big data, combined with accelerating machine learning technology, destined to take over the world? The truth is, nobody knows. But perhaps we all should pay attention, to ensure that the future is a glorious age of information, and not a brave new world.